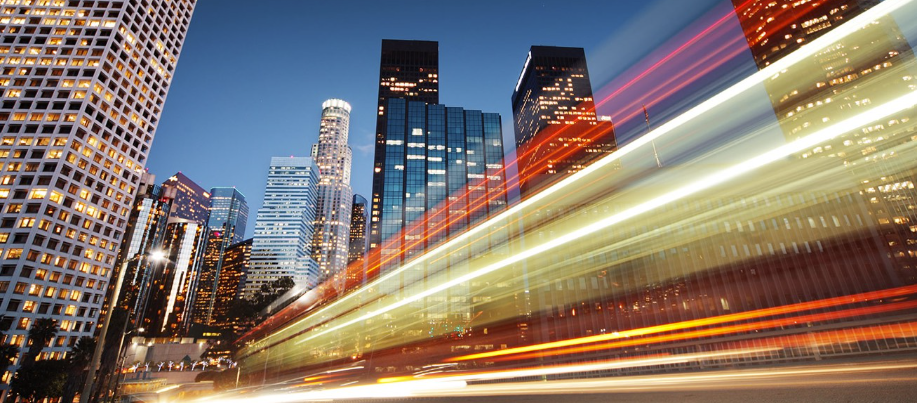
Qlik Compose® for Data Lakes
The quickest route to analytics-ready data lakes.
Speed up and streamline the process of designing, developing, testing, deploying, and updating data warehouses.
Automate data pipelines ready for analytics
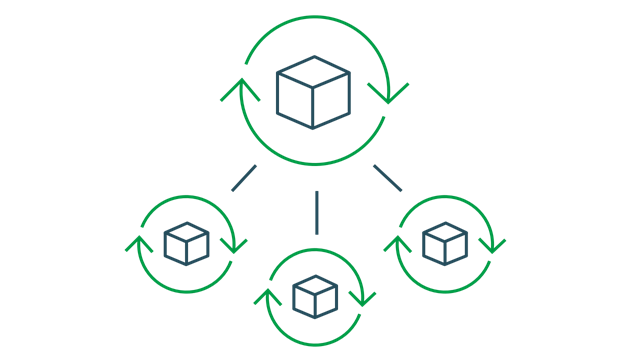
Effortless structuring and transformation of data
Ongoing updates
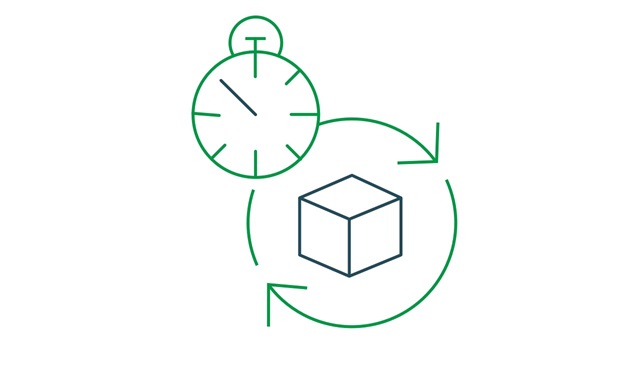
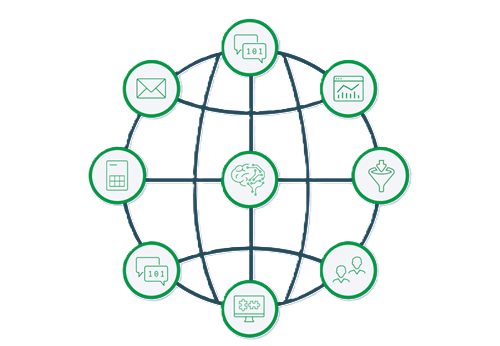
Live Views of data
Create cost-effective, low-latency views of data through the following methods:
- Merge the most recent unprocessed changes in the change table (including the last open partition) during reading.
- Optimize computation by establishing “live views” for both Operational Data Stores (ODS) and Historical Data Stores (HDS) without the need to process changes every time.
Historical data store
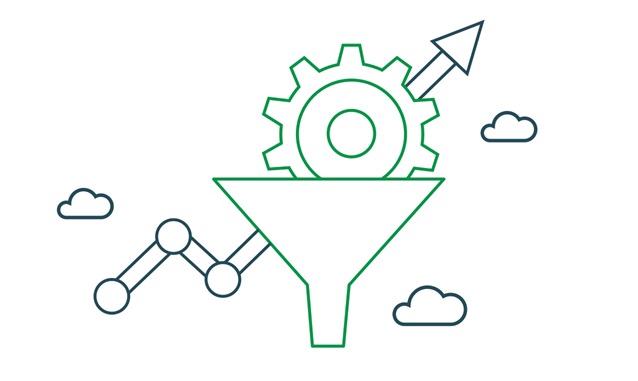